CSULB Professor’s Machine Learning Expertise Contributed to Covid-19 Research
When it comes to Covid-19, conventional wisdom has been that risk factors such as age, obesity, heart issues, and diabetes contribute to worse outcomes. However, according to a new study published in Frontiers in Medicine, the picture may be more complex than that.
A group of researchers from Columbia University Irving Medical Center, University of Pittsburgh, and California State University Long Beach evaluated about two dozen variables from 528 patients hospitalized during the early stages of the pandemic. Using machine learning and artificial intelligence (AI), they found that grouping patients into endotypes, according to underlying biological and physiological conditions, painted a more complete picture.
“One of the questions that has puzzled the doctors is related to the variety of the outcomes that they see when it comes to Covid-19,” said Biomedical Engineering Department Chair Shadnaz Asgari, who helped develop and implement machine learning and artificial intelligence techniques to generate the study’s results. “Sometimes they have a patient who looks healthy and they end up losing them. Sometimes patients with complications survive.”
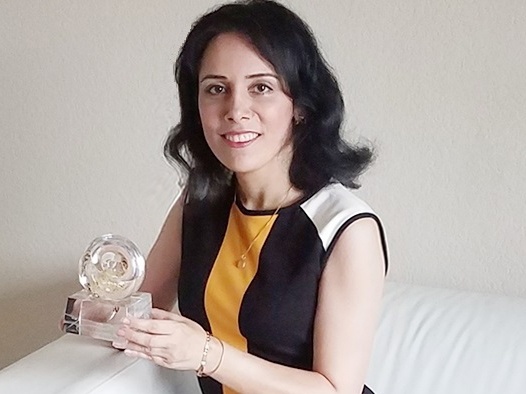
The research’s goal was to come up with criteria to better predict patient outcomes. “That would help the doctors a lot in terms of treatment plans and communicating with families of the patient,” said Asgari, who is also a professor in the CSULB Computer Engineering and Computer Science Department and specializes in using advanced signal processing algorithms and artificial intelligence techniques to deliver practical solutions for biomedical applications.
Applying sophisticated algorithms to data from the 528 patients—including lab values, demographics, outcomes, and treatments—enabled the researchers to divide them into four groups, or endotypes, with particular characteristics.
Endotypes 1 and 2 were comprised of low numbers of intubated patients (1 and 6 percent) and exhibited low mortality (1 and 6 percent), while endotypes 3 and 4 included high numbers of intubated patients (72 and 85 percent) with elevated mortality (21 and 43 percent). Endotypes 2 and 4 had the most risk factors. Endotype 1 patients had low levels of inflammatory markers, low infectious markers, and low degree of excessive bleeding or clotting, while endotype 4 had higher levels of those markers.
“Endotyping of Covid 19 patients is obviously a very hot topic these days from a doctor’s perspective, as it helps them with better patient management,” said Asgari. “The amount of data was big enough for AI and machine learning to come into play and obtain the endotypes.”
An important part of machine learning is selecting the appropriate algorithm to use for a particular dataset. For example, some algorithms are better suited for heterogenous datasets, while others are suited for more homogenous data. Before the algorithm is applied, the data has to be processed to account for things such as missing or inaccurate values. This involves a lot of coding, said Asgari. “Then you have to interpret the results in a form that is clinically meaningful.”
The research indicated that pre-existing conditions alone are not responsible for variable clinical outcomes in Covid-19. “In that sense, our study is eye-opening,” she said, adding that further study may look at the difference in outcomes for the initial Covid-19 virus and its more infectious Delta variant.
Asgari said the collaboration began when she met Soojin Park, an associate professor of neurology at Columbia University, and a neurocritical care physician at Columbia University Irving Medical Center/NewYork-Presbyterian, at intracranial pressure and brain monitoring conference in 2019. They talked about their common interest in brain research. Park had access to a large database, and Asgari’s research specialty of applying machine learning to biomedical data was a perfect fit. So when Covid-19 happened, Park asked Asgari to collaborate on the research project. Additional faculty researchers were invited to join.
“You cannot solve a research problem with one person’s expertise. A real-world problem requires collaboration,” said Asgari.
Using AI and machine learning for clinical decision-making is still in its early stages. Doctors are currently using AI as a supplement to determining patient treatments. But that won’t be the case in the future, when autonomous clinical decision-making will be the norm. “As they become more accurate, you will go to a clinic, someone will ask questions and do tests, and the machine will tell you what treatment makes more sense,” said Asgari. “This will be the future.”